Climate change detectable in daily rainfall patterns, deep-learning model finds
Sep 27, 2023
- Researchers have developed a deep-learning AI model that predicts how global warming is affecting daily precipitation patterns around the world.
- Using the model, scientists found that every year since 2015 daily rainfall deviated from natural variability at least 50% of the time as a result of rising temperatures.
- Research has long focused on climate change’s long-term impacts such as annual temperature increase or precipitation rates.
- However, studies in recent years have observed how rising temperatures are altering daily weather patterns as well.
Scientists have long known that global warming is upending not just seasonal climate trends, but also minute weather patterns on a daily basis. However, establishing a strong link hasn’t always been easy, which means most research has focused on climate change’s impact on long-term trends, such as annual precipitation or monthly temperature increases.
The past few years, though, have seen data and subsequent modeling get more detailed and nuanced. A study published in 2020 in the journal Nature Climate Change delineated how the impact of climate change was detectable from the changes in daily temperature and moisture around the world.
On the heels of that research, and partly inspired by it, another group of researchers took up the task of understanding how variations in daily rainfall might be linked to global warming. Using a deep-learning model, the scientists determined how, on any single day, global warming is impacting, and significantly altering, rainfall patterns around the world.
In their study published in August in the journal Nature, the researchers described how “daily precipitation has now become more variable due to human-induced global warming.” While variability has always been a hallmark of daily weather (think of how often weather forecasts have been off), things like rainfall or snow can generally be narrowed down to a band of probability (hence “a 30% chance of rain,” rather than a definitive declaration of rain).
The model that the researchers developed for their study found that every year since 2015, daily rainfall deviated clearly from this band of natural variability more than 50% of the time as a result of rising temperatures. This, the study concluded, showed that “daily precipitation data represented an excellent predictor for observed planetary warming.”
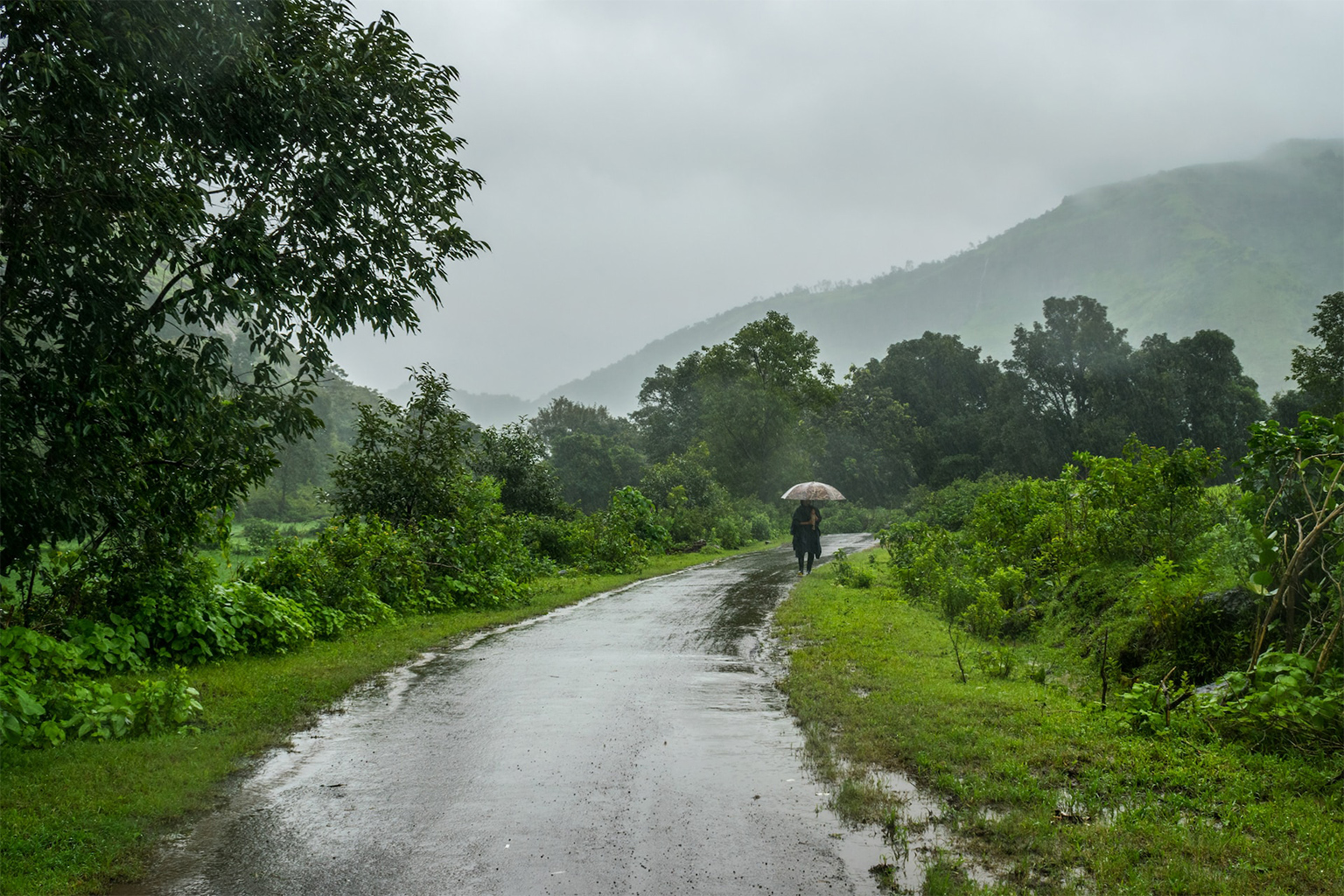
“We were surprised to find that this intensification of global daily rainfall variability is dominated on weather-time scales, periods shorter than 10 days,” study co-author Seung-Ki Min, professor in the climate change research lab at Pohang University of Science and Technology in South Korea, told Mongabay in an email interview. “This means that global warming is indeed changing our daily weather, making it more variable and inducing increased occurrences of extreme wet and dry conditions.”
Monitoring variations in daily precipitation, and why it’s happening, isn’t an easy task. Studying the trend on a short-term scale, and linking it to climate change — a longer-term phenomenon — can get complicated. Daily precipitation might change drastically over the course of a day, which often means that the unevenness in its pattern isn’t always easy to quantify. Additionally, changes in precipitation patterns studied over a longer time are often described in terms of an increase or decrease in a particular month or year. With daily precipitation, which is often erratic and can either increase or decrease in a short span of time, similar ways of measurements often leave out the nuances in the data.
It’s to incorporate these “non-linear” trends in daily precipitation and analyze them more effectively that the team decided to use deep learning, a subfield of artificial intelligence.
“The day-to-day variation is way stronger than the changes in the long-term averages,” study co-author Yoo-Geun Ham, a professor at Chonnam National University in South Korea, told Mongabay in an email interview. “In this respect, we thought deep learning was the best choice.”
The team trained the model to recognize patterns based on data gathered over many years from global climate models. This included daily precipitation maps, along with data on annual global mean temperatures. Once the model was trained, the researchers could feed it global daily precipitation maps, upon which it predicted the annual global mean temperature (AGMT), often considered a good metric for global warming. If the predicted AGMT was found to be higher than what it has been historically, it would not only indicate a change in daily precipitation, but also a link to global warming.
Ham said the model could, in the future, be further developed using the latest image-recognition techniques to improve its accuracy. As extreme weather events become more frequent across the globe, Ham said, the model could serve as a tool to make predictions that could help implement policy measures to mitigate the impacts of global warming.
Banner image: A chestnut-mandibled toucan in the rain. Image by Zdeněk Macháček via Unsplash (Public domain).
Citations:
Sippel, S., Meinshausen, N., Fischer, E. M., Székely, E., & Knutti, R. (2020). Climate change now detectable from any single day of weather at global scale. Nature Climate Change, 10(1), 35-41. doi:10.1038/s41558-019-0666-7
Ham, Y.-G., Kim, J.-H., Min, S.-K., Kim, D., Li, T., Timmermann, A., & Stuecker, M. F. (2022). Anthropogenic fingerprints in daily precipitation revealed by deep learning. Nature. doi:10.1038/s41586-023-06474-x<